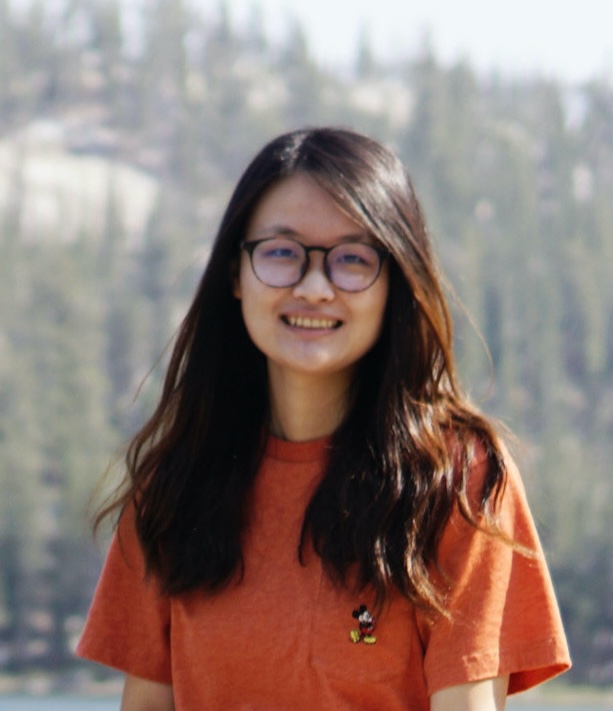
Hi! I am currently a Wojcicki-Troper Postdoctoral Fellow at Harvard Data Science Initiative, also affiliated with Harvard Medical School.
Starting July 2025, I will be an Assistant Professor in the Department of Statistics and Data Science at the Wharton School, University of Pennsylvania. I obtained my PhD in Statistics from Stanford University in 2024, advised by Professors Emmanuel Candès and Dominik Rothenhäusler. Here are my CV, Github and Google scholar pages.
I currently help organize the Online Causal Inference Seminar.
Research interests
I work on statistical problems related to (i) confident deployment of black-box prediction models in critical domains, and (ii) generalization of statistical findings to new contexts. Specifically,
-
Uncertainty quantification:
I think about what guarantees are practically meaningful, how to achieve them with easy-to-use, widely-applicable methods, and applications of uncertainty-aware AI in biomedical discovery and human decisions. -
Generalizability and robustness:
I study the nature of real distribution shifts in practice, models to describe them, and methods to protect against them in generalizing treatment effects, replicating experiments, and learning new decision rules.
News
-
May 2024: Outputs from black-box foundation models must align with human values before their use. For example, can we make sure only human-quality AI-generated medical reports are deferred to doctors? Check out our new paper Conformal Alignment which certifies aligned outputs to deploy with FDR control!
-
March 2024: How to quantify the uncertainty for an “interesting” unit picked by a complicated, data-driven process? Check out JOMI, our framework for conformal prediction with selection conditional coverage!
-
Jan 2024: Interested in making good decisions with conformal prediction? I’ll give a virtual talk “Selecting Trusted Decisions from AI Black Boxes: Correcting Conformal Prediction for Selective Guarantees” at MLBoost (event link, Jan 4, 2024). This is based on our work on model-free selective inference [1, 2].
-
Sept 2023: I’ll be giving a seminar at Genentech Inc. on how to leverage model-free selective inference and conformal inference [1, 2] for reliable AI-assisted drug discovery.
-
Sept 2023: Scientists often refer to distribution shifts when effects from two studies differ, e.g. in replicability failure. Do they really contribute? See our preprint for a formal diagnosis framework. Play with our live app, or explore our data repository! I gave an invited talk about it in the Causality in Practice Conference.
Education
- Ph.D, Statistics, Stanford University, 2019 - 2024.
- B.S. Mathematics, Tsinghua University, 2015 - 2019.
- B.A. Ecomonics (Finance), Tsinghua University, 2015 - 2019.
Beyond academics, I love traveling and photography in my free time. See my photography gallery!